Generative AI is making waves across various sectors, with healthcare standing out as one of the most impacted fields. This technology is reshaping how we diagnose diseases, develop treatments, and interact with patients. By creating new data and solutions from existing information, generative AI is driving significant advancements in medical practice. This article explores how generative AI is transforming healthcare, including its applications, benefits, and challenges.
The Rise of Generative AI in Healthcare
Generative AI, a subset of artificial intelligence, focuses on creating new data and solutions based on patterns learned from existing data. Unlike traditional AI, which primarily classifies or analyzes information, generative AI innovates by producing novel insights. In healthcare, this technology is enhancing diagnostic accuracy, personalizing treatments, and improving patient interactions.
Dr. Eric Topol, a leading figure in digital medicine, explains, “Generative AI represents a leap forward in how we approach healthcare. It enables us to harness data in ways that were previously unimaginable, leading to breakthroughs in both diagnostics and treatment.“
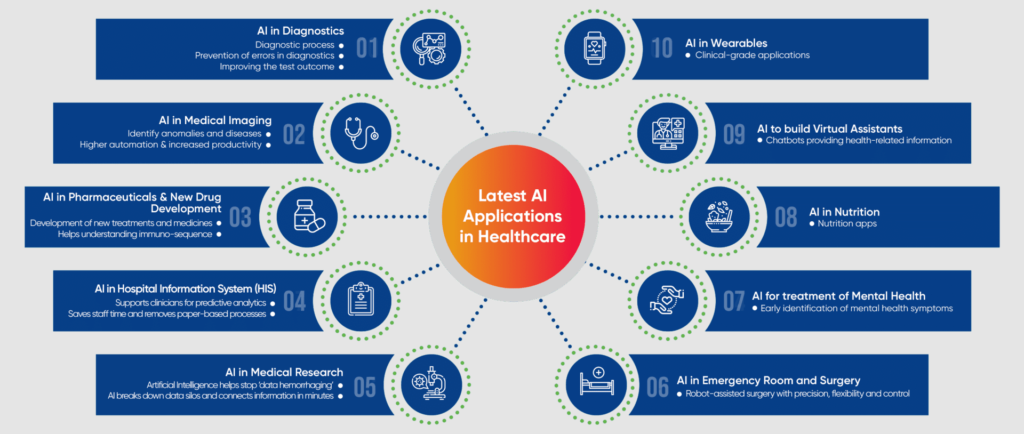
Enhancing Diagnostics with Generative AI
Generative AI is revolutionizing diagnostics by improving both accuracy and efficiency. Traditional methods often depend on manual interpretation, which can be inconsistent and error-prone. Generative AI addresses these issues by analyzing vast datasets and identifying patterns that human eyes might miss.
AI-Driven Diagnostic Tools
Generative AI models are being developed to create advanced diagnostic tools that support healthcare professionals in early disease detection. These tools analyze medical images—X-rays, MRIs, CT scans—to identify abnormalities with greater precision.
For instance, Google’s DeepMind has developed an AI model that detects over 50 eye diseases from retinal scans with impressive accuracy. According to Dr. Demis Hassabis, CEO of DeepMind, “Our AI technology has shown that it can match or even surpass human experts in detecting certain eye diseases. This is a testament to the power of generative AI in enhancing diagnostic capabilities.”

Personalized Treatment Plans
Generative AI is instrumental in devising personalized treatment plans. By integrating data from diverse sources, such as medical history, genetic profiles, and lifestyle factors, AI generates customized treatment strategies.
IBM’s Watson for Oncology exemplifies this application. It analyzes a patient’s cancer profile and suggests treatment options based on a broad database of research and clinical trials. Dr. Andrew Norden, Head of IBM Watson Health, notes, “Generative AI allows us to sift through vast amounts of data quickly, providing oncologists with actionable insights tailored to individual patients. This personalized approach enhances treatment efficacy and reduces the trial-and-error process.”

Accelerating Drug Discovery and Development
The traditional drug discovery process is lengthy and costly. Generative AI is transforming this process by optimizing drug discovery, development, and testing phases.
AI in Drug Design
Generative AI models generate new chemical compounds and predict their efficacy and safety. These models analyze existing chemical data and biological interactions to suggest novel drug candidates.
BenevolentAI provides a notable example of AI in drug design. The company used AI to identify a potential treatment for COVID-19 by analyzing existing drugs and mechanisms. Dr. Joanna Shields, CEO of BenevolentAI, comments, “AI has the potential to revolutionize drug discovery by accelerating the process and identifying promising compounds that traditional methods might miss. Our work on COVID-19 demonstrates how quickly AI can adapt to emerging health crises.”
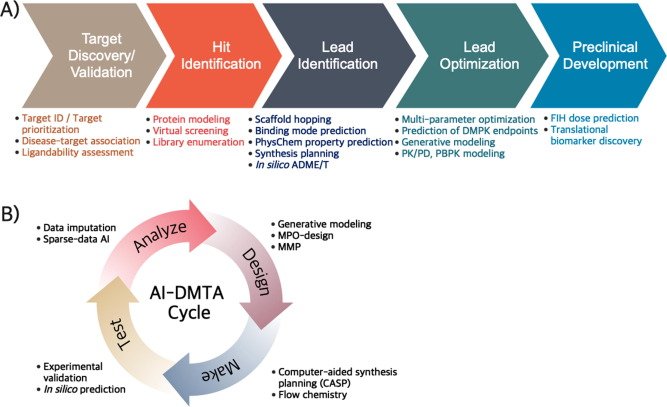
Simulating Clinical Trials
Generative AI is also used to simulate clinical trials, allowing researchers to predict outcomes and identify potential issues before actual trials. This simulation helps design more effective and resource-efficient trials.
Trialjectory’s AI platform exemplifies this approach. It predicts clinical trial outcomes and identifies suitable candidates, optimizing trial design and improving the chances of successful results. Dr. Michael Apkon, CEO of Trialjectory, says, “By leveraging AI to simulate trials and identify ideal candidates, we streamline the research process and improve the likelihood of successful outcomes.”
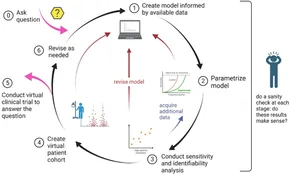
Revolutionizing Medical Imaging
Generative AI is enhancing medical imaging, crucial for accurate diagnosis and treatment planning. AI advancements improve image quality and interpretation, leading to better patient outcomes.
Enhanced Image Analysis
Generative AI improves medical image analysis by enhancing image resolution, reducing noise, and highlighting significant features. This enhancement helps radiologists detect subtle abnormalities, which are critical for diagnosing conditions like tumors or neurological disorders.
Siemens Healthineers has developed an AI-driven imaging platform that enhances MRI scans, making them clearer and more detailed. Dr. Bernd Montag, CEO of Siemens Healthineers, states, “Our AI technology elevates the quality of medical imaging, enabling radiologists to detect even the most subtle abnormalities with greater accuracy. This advancement significantly improves diagnostic precision.”
Generating Synthetic Medical Images
AI generates synthetic medical images for training and validating diagnostic algorithms. These synthetic images simulate various conditions, providing diverse datasets for AI models.
The Medical Imaging Interaction Toolkit (MITK) uses AI to create synthetic images that help develop and test imaging algorithms. This approach ensures that AI models are exposed to a wide range of scenarios, improving their robustness and accuracy. Dr. Hannes H. N. D. Fritsche, Director of MITK, notes, “Synthetic images play a crucial role in training and validating AI models, allowing us to test algorithms under diverse conditions that enhance their reliability.”
Improving Patient Interaction and Support
Generative AI is enhancing patient interactions and support systems, making healthcare more accessible and efficient.
AI-Powered Virtual Assistants
AI-powered virtual assistants are transforming patient interactions by providing real-time information, answering queries, and assisting with administrative tasks like scheduling. These assistants use natural language processing (NLP) to interact with patients conversationally.
HealthTap’s AI platform offers virtual consultations and health advice through a chatbot interface. This technology allows patients to quickly access information and support, improving their overall healthcare experience. Dr. Ron Cohen, CEO of HealthTap, says, “AI-powered virtual assistants bridge the gap between patients and healthcare providers, offering timely information and support that enhances patient engagement and care.”

Supporting Mental Health
Generative AI also supports mental health through virtual therapy sessions, personalized wellness plans, and supportive content. These tools offer tailored interventions based on individual needs.
Wysa is an AI-driven platform that provides virtual mental health support via chat-based interactions. Wysa offers tools for managing stress, anxiety, and depression, making mental health resources more accessible to users. Dr. Julie Li, Chief Scientist at Wysa, explains, “AI-driven mental health tools provide personalized support that adapts to individual needs, making mental health resources more accessible and effective.”
Addressing Ethical and Regulatory Challenges
Generative AI brings significant benefits but also presents ethical and regulatory challenges that must be addressed to ensure responsible use.
Data Privacy and Security
Generative AI requires extensive data, raising concerns about privacy and security. Ensuring compliance with data protection regulations and implementing robust security measures are essential to protect patient information.
Regulations like the Health Insurance Portability and Accountability Act (HIPAA) in the U.S. and the General Data Protection Regulation (GDPR) in Europe set standards for data privacy and security in healthcare. Adhering to these regulations helps safeguard patient data and maintain trust. According to Dr. Megan Ranney, an emergency physician and digital health expert, “Protecting patient data is paramount in the age of AI. Ensuring compliance with privacy regulations is crucial to maintaining trust and safeguarding sensitive information.”
Mitigating Bias and Ensuring Fairness
AI models can inherit biases from their training data, leading to potential disparities in treatment outcomes. Addressing these biases and ensuring fair and equitable care is a critical challenge.
Efforts to reduce bias include using diverse training datasets and implementing fairness algorithms. For example, Google Health’s AI models are trained on a diverse set of medical images to improve accuracy and reduce bias across different demographic groups. Dr. Ivor Benjamin, President of the American Heart Association, remarks, “Reducing bias in AI models is essential for ensuring equitable healthcare. Diverse training datasets and fairness algorithms are key to achieving this goal.”

The Future of Generative AI in Healthcare
As generative AI technology evolves, its potential to transform healthcare will continue to grow. Here are some emerging trends and future prospects.
Integration with Emerging Technologies
Generative AI will increasingly integrate with other emerging technologies like genomics, wearable devices, and the Internet of Things (IoT). This integration will enable more comprehensive and personalized healthcare solutions.
For example, combining AI with genomic data can enhance genetic screenings and targeted therapies. AI-powered wearable devices can continuously monitor health, providing real-time data for personalized treatment and early intervention. Dr. Lisa Su, CEO of AMD, highlights, “The integration of AI with emerging technologies will redefine healthcare, offering more personalized and proactive care through advanced data insights and real-time monitoring.”
Advancements in AI Algorithms
Advancements in AI algorithms will further enhance the capabilities of generative models, leading to more accurate predictions and better decision support. Research into advanced algorithms, such as deep learning and reinforcement learning, will continue to drive innovations in healthcare.
Deep learning advancements, for instance, are expected to improve AI’s ability to process complex medical data, leading to more precise diagnostic tools and tailored treatment recommendations. Dr. Fei-Fei Li, Co-Director of the Stanford Human-Centered AI Institute, states, “Deep learning and advanced algorithms will drive the next wave of innovations in healthcare, making AI models more accurate and capable of handling complex medical data.”
Conclusion
Generative AI is transforming healthcare by enhancing diagnostics, accelerating drug discovery, improving medical imaging, and supporting patient interactions. While the technology offers substantial benefits, addressing challenges related to data privacy, bias, and regulatory compliance is crucial. These steps ensure that the advancements in generative AI lead to equitable and effective healthcare solutions.
As generative AI technology evolves and integrates with emerging technologies, its potential to improve patient outcomes and streamline healthcare processes will expand. By leveraging generative AI responsibly, we can look forward to a future where healthcare is more efficient, personalized, and accessible.
- Exploring C AI: The Next Frontier in Chatting with AI Characters - August 29, 2024
- How to Use Artificial Intelligence: A Practical Guide - August 28, 2024
- Revolutionizing Economics: How AI is Shaping the Future of Financial Analysis and Policy - August 27, 2024